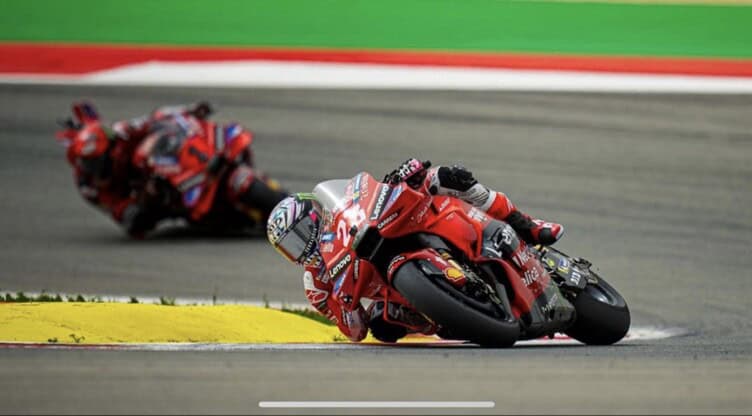
The convergence of dAI with traditional sectors like finance and sports is spearheading a revolution reminiscent of the “Moneyball” approach to baseball management. This philosophy of leveraging undervalued assets and making data-driven decisions is now influencing trading models within the financial sector, with technology serving as the cornerstone for innovation and efficiency.
The story of the Oakland A’s, encapsulated by the line, “For forty-one million, you built a playoff team…You won the exact same number of games that the Yankees won, but the Yankees spent one point four million per win and you paid two hundred and sixty thousand,” underscores a broader trend towards maximizing efficiency and value with minimal expenditure.
This principle is finding resonance in the financial world through dAI and machine learning, as demonstrated by entities like the Voleon Group. This hedge fund exemplifies the integration of cutting-edge data science and AI in finance, leveraging proximity to technological innovation hubs to gain a competitive edge.
AI’s role in transforming financial strategies mirrors the disruptive potential seen in “Moneyball,” with the aim to develop systems capable of autonomously navigating and outperforming the market. Despite historical challenges, the confidence in AI to decode complex market signals and trends is growing, supported by advancements in machine learning and AI technologies.
AWS emerges as a pivotal player in this transformation, providing the necessary infrastructure for the development and deployment of AI-driven trading systems. Scott Mullins, GM AWS Financial Services and Tal Cohen, President Market’s Platform, Nasdaq discuss their collaboration in building the next generation of cloud-enabled infrastructure for the world’s capital markets with AWS.
This enables the creation of sophisticated models that tackle market complexities, facilitating a shift towards data-driven, and efficient trading strategies.
Now I am going to delve deeper into large language models, LLMs and small language models, SLMs.
LLMs and SLMs
The advent of LLMs brought about a revolution in handling and interpreting vast swathes of natural and programming languages. Their capacity for understanding complex language patterns and executing intricate reasoning tasks set a high bar for artificial intelligence applications.
However, the one-size-fits-all approach of LLMs often falls short in addressing queries requiring niche expertise or domain-specific knowledge. This gap has led to the rising popularity of SLMs, designed to offer tailored solutions, better manageability, and lower operational costs.
SLMs stand out by their compact size, fewer parameters, and the reduced volume of training data required. They are engineered to run efficiently on minimal hardware, such as a single GPU, making them an ideal choice for on-premises or on-device applications where security and data privacy are paramount.
Distinctive Features and Applications
The primary distinction between SLMs and LLMs lies in their scale and training breadth. LLMs, with their extensive training datasets, excel in general language understanding and complex reasoning. Conversely, SLMs, with focused training, offer specialized knowledge in particular domains. This specialization makes SLMs particularly valuable in fields requiring specific lingo or expertise, such as legal, medical, or technical domains.
SLMs are increasingly used in diverse sectors, including healthcare, technology, education, and customer service, for tasks like text summarization, sentiment analysis, chatbot development, and more. Their ability to be fine-tuned for specific tasks further enhances their utility, allowing for customized solutions that better address user needs.
Fine-Tuning and Platform Independence
Fine-tuning pre-trained models like Llama-2-13b and available in Amazon Bedrock involves retraining them on a new dataset relevant to a specific domain or task, enhancing their performance for particular applications. This process, while computationally intensive, is made feasible through advancements in hardware and cloud computing platforms such as Databricks, which facilitates model training across various cloud environments.
Hardware Requirements and Data Preparation
Effective fine-tuning requires robust hardware, including GPUs for processing, a powerful CPU for data management, and ample storage. The process begins with data extraction and preparation, crucial steps that determine the model’s performance. Generating question-and-answer pairs from the domain-specific text is a key part of preparing a dataset for fine-tuning.
Challenges and Observations
The fine-tuning journey involves navigating challenges related to the integration of various components, managing hardware resources, and optimizing training parameters. Despite these hurdles, the outcome often justifies the effort, with SLMs demonstrating a remarkable ability to generate domain-specific responses that rival those of their larger counterparts. This capability underscores the potential of SLMs to deliver customized, efficient solutions.
Economic and Environmental Considerations
SLMs offer significant advantages over LLMs in terms of operational costs and environmental impact. Their lower computational requirements translate into reduced energy consumption and operational expenses, aligning with broader sustainability goals. However, the trade-off comes in the form of narrower knowledge bases and the potential for more limited responses in certain contexts.
The Future of SLMs
As AI continues to evolve, the distinction between SLMs and LLMs becomes increasingly nuanced. The specific advantages of SLMs, particularly in terms of customization, efficiency, and security, position them as valuable tools in the AI landscape. The ongoing development and deployment of SLMs across various platforms and industries highlight their potential to complement LLMs, offering a more tailored and cost-effective approach to AI-driven solutions.
The exploration of SLMs, and now Upstage launching this on AWS, from their definition to their applications and the fine-tuning process, reveals a dynamic area of AI research and development. With each advancement, the potential for these models to transform industries and enhance our interaction with technology grows, heralding a future where AI is not only powerful but also precise and personalized to individual needs.